Presenting at the 2023 IEEE 26th International Conference on Intelligent Transportation Systems (ITSC)
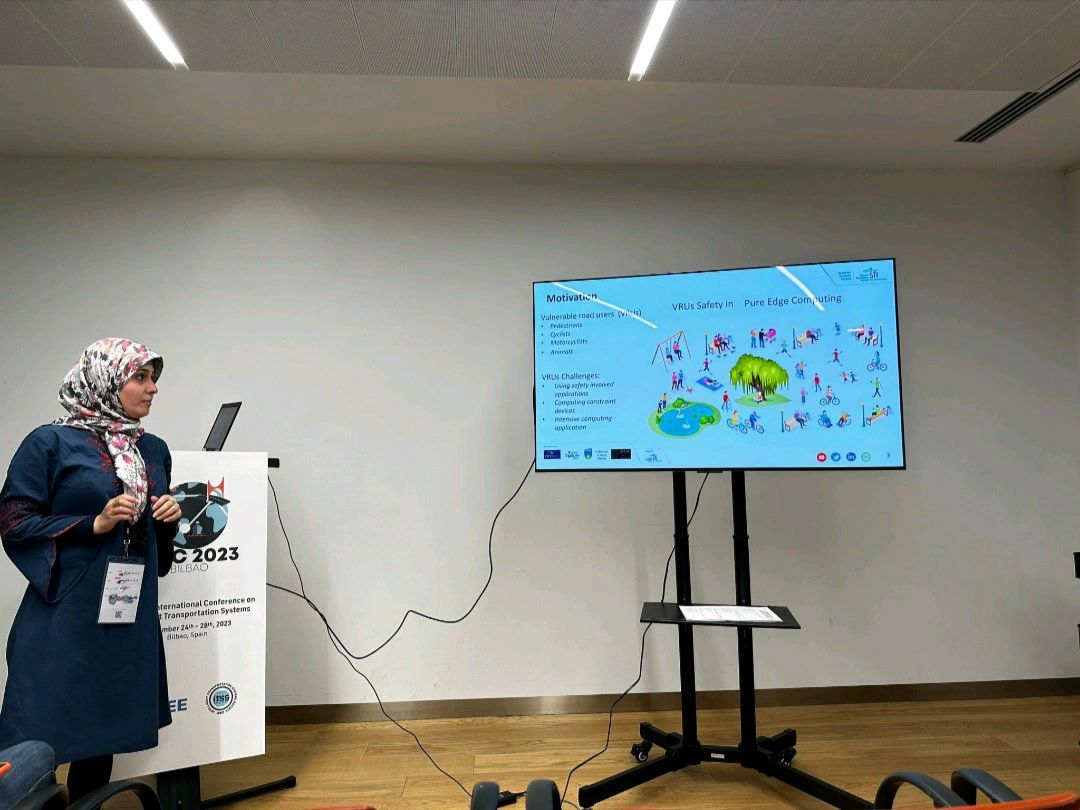
Title: Enhancing VRUs Safety Through Mobility-Aware Workload Orchestration with Trajectory Prediction using Reinforcement Learning
Abstract: Vulnerable road users (VRUs) such as pedestrians, cyclists, motorcyclists, and animals are at the highest risk in the road traffic environment since they move in the environment without any protection. Various applications and architectures that are applicable to Intelligence Transportation Systems (ITS) must be designed by considering this regard. Task offloading is a well-known approach in various ITS applications. Task offloading in edge computing refers to the process of transferring certain computing tasks or workloads from a local device to edge nodes or servers located closer to the device. Orchestrating workload in an environment where both the task generator and destination device can be mobile is challenging while it is crucial for VRUs’ safety. For example, when a user of a blind navigation assistant offloads a task, the success of that task is extremely important. Failure could potentially cause harm or negative consequences. This paper proposes a mobility-aware workload orchestration model for VRUs safety applications. To guarantee a high success rate and reduce the risk of task failure due to mobility, this model uses reinforcement learning to adapt to the dynamic edge environment. This model also employs a heuristic algorithm for device trajectory prediction from basic device location data. In addition, a novel technique is developed for task transferring to avoid task failure of mobile resources. The results show the proposed model outperforms in increasing the task success rate and decreasing the task failure rate due to mobility compared to the baselines.