Presenting at Transport Research Arena (TRA) Conference
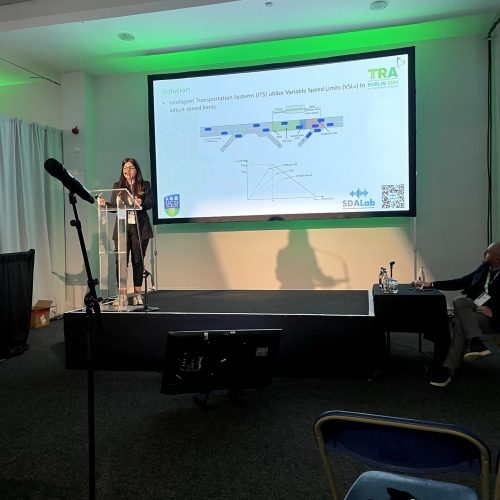
Title of the Paper: Enhancing The Performance of Emergency Vehicles Through Reinforcement Learning Based DVSL Control Systems
Abstract: Variable Speed Limits (VSL) have been used to dynamically control traffic via the adaptation of vehicular speed with the aim to mitigate congestion, enhance safety and traffic flow performance. Recent studies have demonstrated the effectiveness of employing Deep Reinforcement Learning (DRL) techniques for VSL controllers, resulting in improved safety, efficiency, and environmental sustainability on highways. However, the conventional RL models for VSL control systems have not been trained to prioritize and facilitate the movement of emergency vehicles. This paper proposes a deep deterministic policy gradient (DDPG) algorithm which utilizes an actor-critic model by training the DVSL controller and a novel reward function to optimize the time loss of emergency vehicles across a highway weaving section. The results show that the proposed approach outperforms the baseline method with no VSL in terms of the accumulated waiting time, accumulated time loss, average time loss of emergency vehicles and the average travel time of all the vehicles.